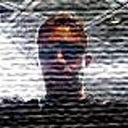

Discover more from Data Machina
Data Machina #238
Non-stop AI Innovation. text-embedding-3. Voyage-code-2. Yi-VL Vision Language. RAG vs Fine-tuning. Google Lumiere. DuckDB-NSQL-7B. InstantID. Dense X Retrieval. DeepMind GATS. Games with AI Agents.
Non-stop AI Innovation Every Single Week. Well yeah, thats’s right: There is no single week without something new, exciting, or amazing happening in AI. This is a selection of interesting, cool stuff that happened in the last 7 days or so:
OpenAI introduced new, faster, and more efficient embedding models. Buried in the blog announcement, it says: “the new embedding models were trained with a technique that allows developers to shorten embeddings without the embedding losing its concept-representing properties.” Well - for some reason- it seems the blog fails to mention that the technique is called Matryoshka Representation Learning (paper, repo), an encoding method for embedding proposed by Google Research in 2022.
Voyage AI announced voyage-code-2, a new embedding model specifically optimised for code-related applications, including semantic code search/retrieval, code completion, and various functions of general code assistants. The model was evaluated on 11 code retrieval tasks, and performs well against OpenAI’s and Cohere’s models.
The team at 01.ai open-sourced Yi Vision Language (Yi-VL), a new, top performant, multimodal model for enabling content comprehension, recognition, and multi-round conversations about images. Yi-VL is based on the LlaVA vision instruction-tuning architecture, and as a few days ago, it was raking first in all the benchmarks for those kind of open source models.
MS Research published a new interesting paper: RAG vs Fine-tuning: Pipelines, Tradeoffs, and a Case Study. This is a must-read for those learning on or building LLM apps that involve RAG or fine-tuning. Which method is better? In which cases? In the paper, the researchers propose a pipeline for fine-tuning and RAG tailored for a specific industry domain, and then they present the pros & cons, and tradeoffs of both methods for multiple popular LLMs, including Llama2-13B, GPT-3.5, and GPT-4. Lots of insights, a great read!
The search for new alternatives to the Transformer & Attention Mechanism, and the Mamba Paper published in December have literally triggered a storm of Mamba-based derivative models, papers and memes too. The new idea is to integrate structured state space models (SSMs) into a simplified neural net without attention or even MLP blocks. Interested in SSMs? Checkout this free, interactive book: State Space Models: A Modern Approach with accompanying Python code. And here are the latest papers on Mamba-based models published in January:
MoE-Mamba: Efficient Selective State Space Models with Mixture of Experts
Vision Mamba: Efficient Visual Representation Learning with State Space Model
Google Research announced Lumiere, a A Space-Time Diffusion Model for Video Generation (paper, demos.) The model produces truly amazing results in: text-to-video, image-to-video, stylised generation, video stylisation, cinemagraphs, and in painting. The innovation here is the introduction of a new Space-Time U-Net architecture that generates the entire temporal duration of the video at once, through a single pass in the model. Checkout the demo video below:
Have a nice week.
10 Link-o-Troned
the ML Pythonista
Deep & Other Learning Bits
AI/ DL ResearchDocs
InstantID: Zero-shot Identity-Preserving Generation in Seconds
DeepMind GATS: A New Approach to Combine Pretrained Foundation Models
MLOps Untangled
data v-i-s-i-o-n-s
AI startups -> radar
ML Datasets & Stuff
Postscript, etc
Tips? Suggestions? Feedback? email Carlos
Curated by @ds_ldn in the middle of the night.
Subscribe to Data Machina
A weekly deep dive into the latest AI / ML research, projects & repos.
Another excellent article. Your substack is one of my primary source of information to understand the AI space. Thank you!